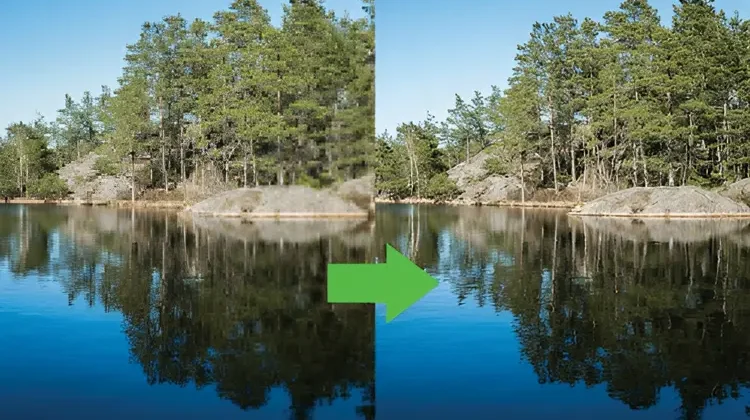
Beyond Interpolation: Exploring Advanced Techniques for Up-scaling Images
Image up-scaling, enhancing an image’s resolution, has become an indispensable tool for photographers, graphic designers, and content creators alike. While traditional interpolation methods have served their purpose, the demand for higher quality and more detailed images has caused the creation of advanced techniques. This article delves into the world of image up-scaling, exploring the limitations of interpolation and showcasing the potential of innovative approaches.
Understanding Image Upscaling
Before diving into advanced techniques, it’s essential to grasp the fundamentals of image up-scaling. It involves increasing an image’s dimensions without compromising its visual quality. Interpolation, the traditional method, calculates pixel values based on neighboring pixels. But, this approach often results in blurry or pixelated images when up-scaling by significant factors.
The Limitations of Interpolation
Interpolation, despite its simplicity, has inherent limitations. When up-scaling images, it struggles to preserve fine details, leading to a loss of sharpness and clarity. Interpolation introduces artifacts, such as ringing or mosaic patterns, which degrade image quality. These drawbacks highlight the need for more sophisticated techniques to discuss the challenges of image up-scaling.
Advanced Techniques for Image Up-scaling
Several advanced techniques have emerged to overcome the limitations of interpolation. These methods use various algorithms and computational approaches to produce high-quality images. Let’s explore the most promising techniques:
Super-Resolution (SR)
Super-resolution (SR) is a class of algorithms designed for image up-scaling. SR techniques leverage advanced mathematical models and machine learning to reconstruct missing information and enhance image details. These methods can be categorized into two main approaches:
- Deep Learning-Based SR: Deep learning has revolutionized image up-scaling, with convolutional neural networks (CNNs) achieving remarkable results. These models learn complex patterns from vast amounts of training data, enabling them to generate correct and pleasing up-scaled images.
- Traditional SR: Traditional SR techniques, such as iterative back-projection and example-based methods, rely on mathematical optimization and image-processing principles. While not as powerful as deep learning-based approaches, they can still produce acceptable results in certain scenarios.
Image Prior-Based Up-scaling
Image prior-based up-scaling uses prior knowledge of image characteristics to guide the up-scaling process. This approach leverages information, such as edge preservation, noise reduction, and texture synthesis, to improve image quality. By incorporating these priors, up-scaling algorithms can generate more realistic and appealing results.
Example-Based Upscaling
Example-based up-scaling, also known as patch-based or dictionary-based up-scaling, searches for similar image patches in an extensive database of high-resolution images. By matching and replacing low-resolution patches with their corresponding high-resolution counterparts, this technique can enhance image details.
Hybrid Approaches
Combining multiple techniques often leads to superior results. Hybrid approaches integrate different methods to leverage their strengths and mitigate their weaknesses. For example, combining deep learning with the image prior can produce up-scaled images with exceptional detail and naturalness.
Evaluating Up-scaling Techniques
Assessing the performance of different up-scaling techniques is crucial for selecting the best method for a given application. Various metrics can be used to evaluate image quality, including:
- Peak Signal-to-Noise Ratio (PSNR): Measures the difference between the original and up-scaled images in terms of pixel values.
- Structural Similarity Index (SSIM): Evaluates the perceptual similarity between images by considering luminance, contrast, and structure.
- Visual Quality Assessment: Human perception plays a vital role in image quality evaluation. Subjective assessments can provide valuable insights into the effectiveness of up-scaling techniques.
Applications of Image Up-scaling
Image up-scaling has a wide range of applications across various industries:
- Photography: Enhancing low-resolution images to create high-quality prints or digital enlargements.
- Video: Improving video resolution for better viewing experiences on high-definition displays.
- Medical Imaging: Enhancing the clarity of medical images for diagnosis and analysis.
- Remote Sensing: Improving the spatial resolution of satellite images for mapping and environmental monitoring.
- Digital Art: Upscaling digital paintings and illustrations for large-scale prints or displays.
Case Studies in Image Upscaling
To illustrate the power of advanced image up-scaling techniques, let’s examine some real-world case studies:
Case Study 1: Restoring Historical Photographs
Historical photographs often suffer from low resolution and degradation due to age and storage conditions. Image up-scaling can be instrumental in restoring these precious artifacts. By applying deep learning-based super-resolution techniques, researchers have successfully recovered lost details and enhanced the clarity of old photographs, bringing history to life.
Case Study 2: Enhancing Medical Imaging
In the medical field, image clarity is paramount for accurate diagnosis and treatment planning. Image up-scaling can significantly improve the quality of medical images, such as X-rays, MRIs, and CT scans. By revealing finer details, these techniques can aid in the early detection of diseases and assist in surgical planning.
Case Study 3: Gaming and Virtual Reality
The gaming industry demands high-resolution visuals for immersive experiences. Image up-scaling can be used to enhance game textures and environments, providing players with a more realistic and detailed gaming world. Additionally, up-scaling techniques can contribute to the development of higher-resolution displays for virtual reality headsets, delivering sharper and more immersive experiences.
Case Study 4: Satellite Imagery
Satellite images are essential for various applications, including weather forecasting, disaster management, and environmental monitoring. Image up-scaling can improve the spatial resolution of satellite imagery, allowing for more accurate analysis and detection of changes over time. For example, up-scaling agricultural satellite images can help farmers monitor crop health and optimize resource management.
Challenges and Future Directions
While image up-scaling has made significant strides, several challenges remain. These include:
- Preserving Fine Details: Accurately reconstructing fine details, such as hair, textures, and small objects, remains a challenging task.
- Computational Efficiency: Deep learning-based methods can be computationally intensive, limiting their real-time applications.
- Data Availability: Sufficient high-quality training data is essential for developing effective up-scaling models.
Despite these challenges, the future of image up-scaling is promising. Ongoing research and development are focused on addressing these limitations and exploring new possibilities. Advances in hardware, such as specialized AI accelerators, will contribute to faster and more efficient up-scaling algorithms. Additionally, the integration of image up-scaling with other image processing techniques, such as noise reduction and color enhancement, holds the potential for even more impressive results.
Conclusion
Image up-scaling has come a long way from simple interpolation techniques. Advanced methods, particularly those based on deep learning, have demonstrated remarkable capabilities in generating high-quality, detailed images. As research continues to progress, we can expect even more impressive results in the future. By understanding the strengths and limitations of different up-scaling techniques, users can select the most appropriate method for their specific needs and achieve optimal image quality.